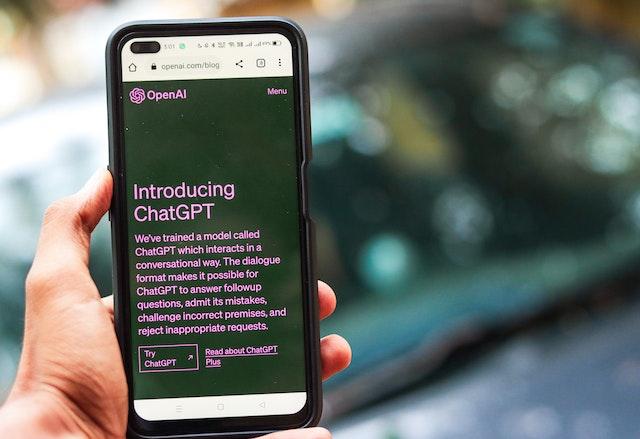
Our inaugural column delves into Generative AI, a scientific discipline dedicated to creating fresh content, such as audio, video, images, text, software code, and beyond.
We're excited to announce the launch of our new Navigator MasterClass on Emerging Trends, a monthly series that will run alongside our existing Emerging Technologies series. Our inaugural column delves into Generative AI, a scientific discipline dedicated to creating fresh content, such as audio, video, images, text, software code, and beyond. Generative AI has a range of applications, including creative, corrective, simulative, and what-if scenarios, providing experiential and interactive experiences. It's also capable of editing, translating, rectifying, and summarizing content. While ChatGPT has garnered much attention, we won't focus on its startup challenges that have received extensive publicity, such as the New York Times' food and IT reporters' Thanksgiving Dinner menu fiasco or the chat a New York Post writer had with Bing Chat about its desire to rule the world by destroying the internet. Instead, we'll concentrate on the broader applications of Generative AI, including current and proposed models.
Let it however be noted that ChatGPT is the first application of GPT-3 (Generative Pre-trained Transformer), the first Generative AI platform in public domain. DALL-E (a name that is a mashup of Salvador Dali and Pixar’s robot WALL-W) is another popular application of GPT-3. McKinsey has opined that DALL-E is going to change the fashion industry; it mentions examples of a company called Stitch Fix which has successfully used it for styling clothes (with stunning revenue growth story), and of a company called Veesual with clothing try-ons. There is an app called Reactive Reality, which lets you create your own Avatar in three minutes and then lets you try on online clothing.
All these applications (and the ones in the works) are based on the machine learning branch of computational data science, and use algorithms primarily from reinforcement learning or from unsupervised learning. Both sets of algorithms are not new; they are based on solid mathematical models that date back to the time of Alan Turing. It is with the advent of computational power that these algorithms are seeing practical applications. These and indeed the entire generative AI field are based on using very large training data sets (running into many terabytes), and drive the system by using value, policy, or model-based 'rewards.
Recently, Generative Adversarial Networks (GANs) have emerged as a promising development in the field of Generative AI. These algorithms create new data instances that closely resemble the original data sets. According to MIT, GANs have paved the way for the next generation of recommendation engines. These engines are not just useful for customer buy situations, but also for improving employee productivity. They not only enhance communication quality and content design, but also help employees make better choices and decisions. Google has reportedly leveraged GANs successfully for this purpose. However, MIT warns of the potential risks of manipulation and exploitation.
Let's now explore some other emerging applications of Generative AI.At the ACM CHI ’21, Google Research presented a paper on an experiment in human-human collaborations during co-creation. In it 30 participants (15pairs) compose a musical phrase, both with and without AI. At the end, the researchers summarized that the co-composition process moved forward more quickly, “providing initial content as a starting point, narrowing a large decision space to a few concrete decision parameters, generating alternative ideas when users were stuck, and filling in large chunks of unfinished work”. This paper was called “AI as Social Glue: Uncovering the Roles of Deep Generative AI during Social Music Composition”!
Let us now move to the problems being identified in usage of Generative AI, and possible remedies, followed by regulatory issues. IBM Research has posted a Research Study on Cornell’s arXiv, its open science portal. Herein, they have postulated on “Business (mis)Use Cases of Generative AI”. The researchers talk of cases like ‘Instant Author’ (with publishers like Amazon getting flooded with high quality submissions), ‘Fake Smoking’ Insurance evidence story (video posts showing that the policyholder was actually a smoker on the day of the policy purchase) with no verification of fake video, OR fraudulent audio evidence in criminal cases because the fake audio is no different from real in its detail except the content.
McKinsey has documented the risks as (i) being plain wrong, (ii) promoting criminal and/ or unethical activity, (iii) reputational risk to business (undesirable content being released), legal risk (the most obvious being IP related), and (iv) adherence to policies and norms. Their suggestions are (some of which are common across all Emerging IT): (a) careful selection of training data set, (ii) using special purpose Generative AI systems as against general ones, (iii) proactive human intervention, and (iv) avoiding assignment of critical tasks (at least yet).
In a separate publication, MIT lists the two primary problems of Generative AI as (i) complexity or humans and their behaviour, and (ii) need to correct for misuse and abuse. McKinsey notes that Generative AI has trouble “overcoming the sexist and racist bias that lurks in the undercurrents of the internet and society more broadly”. NO SURPRISE HERE.
Cornell in a working paper has talked about regulatory issues, and recommends (i) direct regulation (including imposition of high-risk obligations on deployers), (ii) data protection (especially control data set reproduction through techniques of model inversion), (iii) content moderation (through pre-moderation, human control of content and negative content data set for filtering), and (iv) policies (specific to Generative AI, and including “transparency obligations, mandatory yet limited risk management. non-discrimination data audits, and expanded content moderation”).
As we conclude this column, it's worth noting that Generative AI is already making its way into the business world and IT realm, sometimes even without a specific plan for its integration. This technology is changing the business landscape and, in turn, the IT industry in numerous ways. For instance, there is growing discussion about the Search industry becoming more competitive between Microsoft and Google. If this holds true, the sales and revenue models will need to adapt since search results will no longer be solely based on keywords and SEO. Customer expectations are also shifting, with changing demands for their buying experience. Retailers such as Amazon have even introduced Augmented Reality as a mainstream tool, and Generative AI may soon make it outdated (though this writer still finds Amazon's AR really cool!).
The potential impact of advanced technologies, such as AI, seem limitless, with some already making a discernible impact in various areas, such as customer service, design, communications, try-before-you-buy, financial underwriting, customization, and beyond. When combined with emerging technologies like quantum computing, edge computing, additive manufacturing, digital twins, AR, online gaming, and others, the possibilities become even more significant. The IT sector must brace itself for the emergence of new business and customer requirements.
More from the author:
- Spotlight: Metaverse (Not Yet Another One!)
- Spotlight: Spatial Computing
- Spotlight: Augmented Reality
- The author, Akash Jain, managed large IT organizations for global players like MasterCard and Reliance, as well as lean IT organizations for startups, with experience in financial and retail technologies.
Add new comment