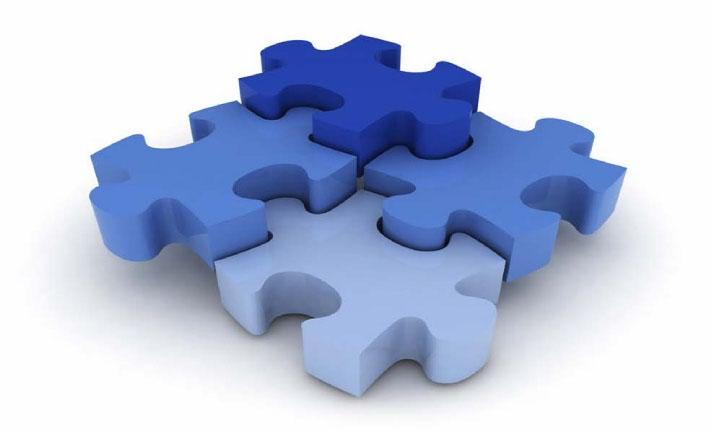
Insights from IBM’s Intelligent Business Strategies states that the type of big data analytical workload may be on structured data taken from a data warehouse or from other data sources (e.g. operational transaction systems) for the specific purpose of doing complex analysis on that data. This may be needed so that power users can mine data to produce predictive models for use in every-day business operations. An example would be to build models that score customer risk. Predictive and statistical models can be built for deployment in database or in real-time operations.
These models may be for use in recommendation services so that the same recommendations are used across all channels of the business that a customer can interact with e.g. website, contact centre, sales person, partner, kiosk and physical outlet (e.g. branch, store). Another example would be to support detailed statistical analysis on spend data to produce models that predict when spending is likely to exceed budget to issue early warnings that keep spending under control.
Some vertical industries are investing heavily in complex analysis to mitigate risk
Oil and Gas provides another example of complex analytics in the area of well integrity, which is very important in managing environmental risk, health and safety and maintaining production volumes. Disasters can be very damaging to corporate brands and so it is often the case that detailed data from multiple files associated with many individual wells are loaded into an analytical DBMS to look for possible problems and to compare readings across wells. It may also be the case that there is a need to contrast actual well data against seismic data to compare actual geology against simulated geology taken from seismic surveys. This may lead to better identification of drilling opportunities.
Add new comment