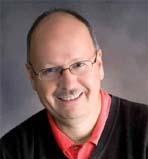
John G Schmidt, VP of Global Integration Services at Informatica, constantly advocates the business potential of emerging technologies to his customers. In a conversation with N Geetha, Schmidt explains how to harness big data with lean data management techniques
Can you elaborate on the influence of big data, and how much sense it makes to IT managers at this point of time?
The industry term big data means different things to different people depending on who you are asking and it is manifested in different forms. However, the basic concept of big data as we perceive is enterprise information in all its formsunstructured, structured, and semi-structured which is becoming massive and as a result, more difficult to manage.
For instance, if you look at public sector, in particular government organisations, they have huge information and data flow, which is cumbersome to manage. This is big data. The telecom companies for instance generate phone bills and massive amount of data transaction happens with the bills monthly billing data running into 170 pages. Big data is the confluence of three mega trends: big transaction data, big interaction data and big data processing. IT heads play a pivotal role in implementing effective data management strategies to address this trend. Big data opens up tremendous new possibilities for government organisations in terms of gaining fast and multifaceted insights that can improve public sector capabilities. This holds good for all the verticals, as IT heads can leverage big data trends to business advantage.
I would advise IT heads to harness big data with lean data management strategy, which can definitely bring greater business advantages.
Please elaborate on how IT managers can leverage the lean data management strategy.
With the increasing shift towards the virtual world, even for simple functions, with the increasing online and mobile transactions with the advent of cloud computing one thing is clearbig data is here to stay. However, while the data is adding up to huge amounts, one pertinent question that arises is, are organisations able to reap as much from this data as they should? In a competitive climate that demands almost 100 per cent efficiency, organisations today are making constant efforts to maximise the return on their data. The organisation that succeeds in such a climate is one that is able to integrate its data in such a way that it minimises wasted effort, provides fast just-in- time delivery and engages workers, who clearly understand their role in the entire process and are empowered to drive improvements. Lean data management strategy is used to harness big data to create opportunities.
Lean Integration represents such a refined phase of data integration and can take the company a long way to this level of efficiency. Being more specific, data can be managed in a lean or non-lean way. Inefficient processes, high work-in-process backlog, slow batch-oriented delivery and executives who are constrained in narrowly defined tasks characterise a non-lean operation. Lean Integration has been shown to generate up to 90 per cent reduction in project lead-time, 50 per cent gain in integration team productivity and significant and continuous improvements in project quality. It means agility for the business, decreased risk for senior IT executives and lower cost for IT developers and managers. With numbers like these, any organisation has a lot to gain in terms of lean integration.
How do you see the big data challenge across industry verticals?
The challenge of the increase in data deluge is invading every sector. Despite the use of the most sophisticated data management tools, every organisation is going through the challenge of data wastage. On an average in every company, about 50 to 70 per cent of the data can be termed as non-accessed data. The legacy systems that are not being used or have not gone through the migration process, also adds to data wastage and unstructured data category. Ninety per cent of the data warehouses are not actively accessing data residing in it and in some cases, it is not metered appropriately.
How does the lean data management strategy work?
We observe that most data landscape across organisations is fertile with opportunities to improve performance across multiple domains, yet riddled with the pitfalls posed by rising data volumes, complexity, diversity and velocity. Lean data management addresses the challenges of data silos and ensures that all data is trustworthy, actionable and authoritative. This is possible as the lean data strategy proactively does knowledge sharing, and facilitates co-creation and interaction between the different business and user groups so that it is effectively used.
Can you elaborate on your strategy to help customers harness big data with lean data management?
As a strategy, we recommend our customers to use certain tools and data integration technologies to measure the wastage of the data in an operational system. Every data is analysed and by combining large-scale transactional data with new interaction data and taking advantage of lean data management practices, we enable customers to leverage existing resources to reduce costs while improving the response times and quality.
Lean data management takes many forms how lean principles can be applied to applications, data warehouses, and portfolio management to maximise the return on data while managing big data growth and its associated costs.
What technologies can customers look at to address big data challenges as part of the lean data management strategy?
Most common and recommended practice is to archive the data in a compressed format. Mostly, sectors like banking and healthcare that are bound by certain mandatory regulations would need to compress the non-active data. Banking, healthcare and other sectors have been using business analytics to determine the structured and unstructured data and analyse data quality, besides driving operational efficiency. Technologies like data de-duplication are being looked at to compress data. ETL is another tool which is being evaluated to derive value of the existing data and gain insights into the data lifecycle. Customers are evaluating master data management (MDM) tools to integrate with CRM packages for consolidating the data.
How are the big data related solutions addressing customer issues?
Our customers, spread across travel, logistics, healthcare, manufacturing, etc, had huge big data challenges. One of the logistics companies had the business need to reduce the cost incurred on the gas and increase operating margins, while providing reliable data for accurate decision-making. We recommended solutions around data quality, identity match option, and data explorer to create a single, unified development environment for trusted and high-quality data. These solutions enabled the company to increase data accuracy and accelerate implementation. The data explorer helped the customer to investigate, discover and map any data record from any source, for reliable and trusted data. Using these solutions, the customer could save millions annually in truck idle time using a unified, reusable data quality environment. With the deployment of the solutions, the new enterprise data warehouse may save between $9 and $20 million over two years. The interesting aspect is that the solution was deployed at a manufacturing company in four and a half months with a team who had previously been unfamiliar with data quality solutions.
There have been reduced emissions which supported the organisations environmental commitments. The customer could see a comprehensive, accurate picture of the content, quality and structure of its enterprise data. The key benefit of the data management solution was to solve business problems faster by empowering the business to participate in data quality process.
Manufacturing companies are increasingly looking at lean data management solutions, as there is a business need to respond more quickly to market change, analyse cost data, and increase efficiency.
One such Indian customer, Asian Paints, used analytical solutions to create a customer user interface to create a shared product-costing system for the companys portfolio of paint products. We helped customers integrate raw material, BOM and other dataregardless of its source or structure, including the deployed SAP, Infor Optiva and i2 (now JDA) environments. The solutions helped in reconciling discrepancies in the way Asian Paints different systems structure data, thereby maintaining a consistent view of the product-costing data across all systems.
Asian Paints saw reduction in time needed to run its product-costing model from 24 hours to 20 minutes on using shared model of the product-costing system.
INFA claims cloud as the fastest growth driver that can address big data challenges. Can you substantiate the statement?
In fact, the cloud model is not a challenge for IT heads. It is enabling them to understand the data challenges better and helping them put up a cloud integration process and allowing them to come up with new offerings.
For instance, Amazon has come up with the best data integration platform on the cloud model. The model that Asian Paints evolved, the product-costing model, is also offered as a cloud integrated shared services model.
Add new comment