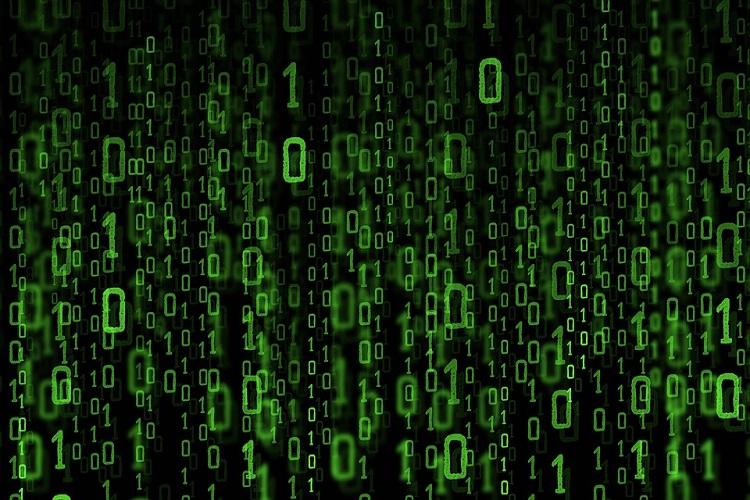
Businesses across the world are collecting massive amounts of data on a daily basis from different kinds of data sources, including telemetry and IoT devices, consumer and business data and even social media. As data becomes increasingly integrated into the way we run our lives and businesses, it is extremely critical how enterprises handle the rapid data growth and manage diverse data to extract maximum business value and to come up with solutions that have real-life implications.
In order to succeed and maintain a competitive edge, organizations are using these data – both historical and real-time - for deep learning and analytics. The insights can be used for improving utilization of resources, cost optimization, one-to-one marketing, promotions and developing new product ideas.
Data lakes: The most important data management infrastructures of tomorrow
A data lake is a repository of structured and unstructured data that enables one to derive insights for their business. One can experiment, explore, and iterate very quickly to build use cases and models on the data lake platform. Data lakes bring data from different data sources together under one umbrella, and since it stores both raw and processed data, it helps support various analytics and Machine Learning (ML) use cases.
Data lakes thus today have emerged as one of the most important data management infrastructures and are being adopted widely across the industry. The basic requirement for a data lake is to allow real-time ingestion and historical data storage. This allows the data to be accessible to data scientists through analytics and deep learning mechanisms. Data lakes also have to layout the real-time and historical data in the right data format so that it can be easily and quickly accessed by the user for analytics, aggregation and time-series queries.
For example, cloud-based services could provide predictive analytics and proactive support to optimize operations across the customer’s hybrid cloud. This is achieved by collecting billions of data points from the many telemetry services. These data points are stored in a huge data lake – whether they are on premises in a local datacenter or on the cloud. Deep learning algorithms are then executed on this data lake right in the cloud.
Deep learning framework
Data scientists and engineers analyze the data using the latest ML and AI techniques. This analysis offers the most robust, actionable intelligence, and data is presented in ways that enables businesses to make smart and fast decisions.
The platform provides a framework where developers can build and test data science and ML algorithms quickly in the data lake. To run the deep learning algorithms, a huge amount of computing power is required. Since on-demand compute can easily be bought in the cloud, the hybrid cloud deep learning algorithm can be executed in the cloud too. Once the algorithms are well tested, the data can be moved from or to the data lake to a cloud-based data storage solution.
This solution lets you use multiple industry-leading clouds (hyperscalers like Amazon, Azure and Google) and maintain complete control over your data on dedicated storage systems from such a data solution.
The hyperscaler clouds are used to deploy micro services that compute or act on the data and make results available to applications or users through the Application Program Interface (API).
Businesses often use such analysis for identifying trends and forecasting and to enhance the efficiency of their infrastructure. It can also help in avoiding or navigating future problems by alerting businesses of similar issues from the past.
The introduction of the data lake platform has led to the democratization of data through self-service analytics and has enabled users to explore data more freely.
Data from other curated data sources can be blended with newly explored data in the data lake for advanced analytics and visualizations. Data lakes have enabled agility for the delivery of innovative solutions at several enterprises such as our own. Users can quickly deliver solutions, get feedback, and iteratively refine the solution before production deployment.
Data lakes can provide invaluable insights through a variety and volume of data, including technologies, such as SPARK, SPARK ML Lib, R, Python, Scala and Akka to enable development, deployment, and execution of ML algorithms.
They can contain valuable insights from customer environments, such as workloads, risk prediction and utilization. This data can be used for understanding customer consumption patterns and uptake of new features and technologies in the field. It can be further used for data driven product feature deprecation and bug impact assessments in field. These analytics also enable to identify the staff to be trained for new features and counter the everyday challenges in the field.
The cost of data lakes and subsequent ROI
With the volume of data growing day by day, the question of data lake sustainability arises simultaneously. Data lake expansion comes at a huge cost; to keep even two years’ historical data, one would need a very big Hadoop cluster. And businesses will not want to get rid of all the historical goldmine data which is allowing deep learning and data analytics that support their data economics vision.
There are, however, ways to cut some costs around data lake expansion. Data lakes costs involve three major cost components: Storage cost, compute cost and license cost. It needs decoupling of the processing framework from the data storage framework which can be achieved by moving to Spark on Shared Files Systems —Spark as computing framework and Shared Files Systems for storage.
Cost optimization of data lake cluster can be achieved by segregating it into data ingestion (ingestion cluster) which is compute-intensive and data consumption (analytics cluster) which is storage-intensive and on-demand compute-intensive.
Data ingestion cluster can be developed based on event-based architecture using Spark, Kafka and Shared Files Systems without using Hadoop. Data analytics cluster needs to follow best practices for data organization. Parquet columnar storage can be used to reduce storage requirement, and it can also enable fast execution of analytics queries.
In fact, it is possible to reduce the number of Hadoop nodes of the ingestion cluster by around 60% by moving the ingestion pipeline from batch processing to event-based processing using various tools.
With data lakes, new business insights and data use cases which were previously considered unfeasible, are now achievable. Companies can now obtain a complete view of their customers, extend the life of their enterprise data warehouse, or achieve a new level of operational intelligence. It not only helps companies to exploit the potential of different data types but also makes legacy systems more efficient by offloading capacity to the newer, more flexible infrastructure.
The author is Senior Vice President, Quality and Manageability Group (QMG) and Managing Director, NetApp India
Add new comment