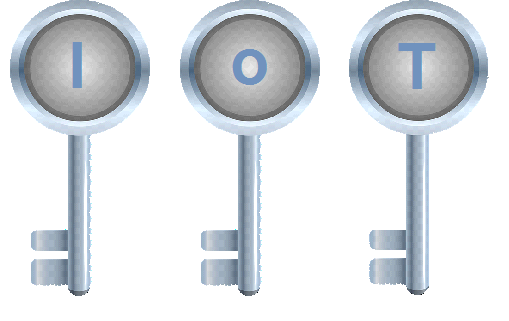
With the rise of low-cost sensors, ubiquitous connectivity and massive data volumes, the “Internet of Things” promises to change the world. We’ve all heard predictions about the billions of dollars and billions of things that will make up this mega-trend by 2050, but that doesn’t tell the full story. Unlocking the true potential of IoT will require overcoming data challenges more so than problems surrounding the “things” themselves.
These data challenges are best described as a “last mile” problem, from the challenges of extracting data from devices, machines and remote platforms to those of interpreting it to drive productivity and peak performance. Whether we’re talking about a connected home, a piece of wearable technology or an industry-scale solution, there’s often a disconnect between collecting new data and actually exposing the information mined in a way that can be deeply understood and explored.
Here are three keys to overcoming these hurdles and taking IoT over the finish line:
1. Interactivity: Smartphones aren’t just instrumental in the Internet of Things but actually offer a compelling analogy for one of its hurdles. Think back to when Steve Jobs first introduced the iPhone to the world. He contrasted the revolutionary new “giant screen” against the standard buttons on phones. His argument for the innovation was that every app needed its own screen and user interface. As he put it, “buttons and the controls can’t change. They can’t change for each application, and they can’t change down the road if you think of another great idea you want to add to this product.”
A similar conundrum applies to analytics. Every question we ask of data needs its own chart and its own visual perspective -- and this is especially true when it comes to the exploding amounts of sensor data that form the foundation of IoT. Unfortunately, most IoT applications ship with “one-size-fits-all” views, perhaps better referred to as “dead-end dashboards.” They answer a pre-determined set of questions, deemed worthy of answering by a small clan of “experts” – whether that means the health experts behind FitBit or the engineers behind GE’s Predix platform.
To realize the full potential of the Internet of Things, tools need to be far more flexible, letting users sculpt and mould data in different ways depending on a user or organization’s needs. Interactivity, drill-ability and sharing are crucial to making IoT data useful without requiring a huge data project. Ideally, users will be able to have casual and in-depth conversations with their data and with other data explores so they can uncover all sorts of permutations and sometimes even reveal patterns they didn’t know existed.
For example, you may be able to use an IoT application that looks at the historical activity data of a broken engine, gas turbine or locomotive to predict what conditions lead to failures and how often a failure event is likely to happen. But what if you wanted to look at the parts that fail the most? And understand which factories manufactured these parts? And know when? And learn what suppliers caused the most issues? That’s where interactivity and share-ability are key.
2. Integration: These in-depth questions are closely related to the second key to IoT success: integration. It’s not just interactive data analysis that will provide answers; it’s also combining IoT data with additional context.
Let’s start with a consumer example: You want to comb your Fitbit data for a possible link between your exercise regimens and sleep patterns. You want to know
- How does physical activity during the day impact my sleeping patterns
- Do I perform better when I have had ample sleep?
The native dashboards in Fitbit only allow you to analyse fitness data in isolation. But if you export data, you can combine that info with other info, like data from tracking your physical activities as well as your food intake, body measurements, and sleeping patterns. An export may not be ideal, but it’s sometimes the only way to broaden the scope of analysis.
Now, instead imagine uncovering enterprise-level insights by blending disparate data. Sensors embedded in a jet engine can help us predict when it might need service. It could help us pre-empt failures and save billions of dollars… and by integrated it without info, it could also help us know our savings compared to our projected budget by product and region, for instance.
3. Iteration: The concept of exporting data (and the fact that it’s not ideal) brings us to an important final point: We live in a world where “perfect data” is increasingly becoming an oxymoron. However well the data may be stitched, it’s ever so likely that it’s stored in a source you can’t connect to. The data may also be missing some key elements critical to answer your questions, or is formatted in a way that’s less conducive to deep analyses. These drawbacks apply to IoT applications as well, especially when there are no consensus on standards and protocols to support device interoperability.
Rather than having bad or incomplete data paralyze our business, though, we must work with what we have and iterate toward the right answers. As you iterate, you learn to separate the “good enough” data from the really bad data. “Good enough” data is usually sufficient to answer most if not all questions directionally. Moreover, better understanding of data gaps leads to better data. It’ll help you fix process issues that’ll improve how your data is captured and ingested -- and help us all move one step closer to the IoT finish line.
The author is country manager, India, Tableau Software
Add new comment