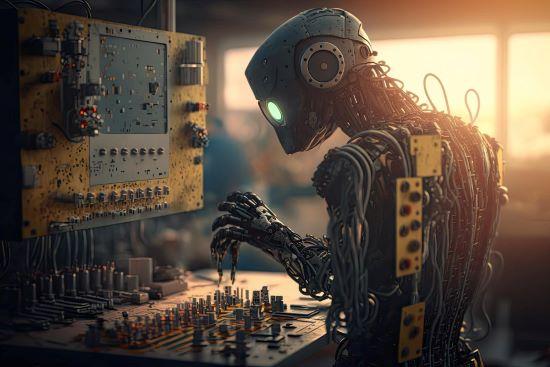
We launched the Navigator MasterClass on Emerging Trends a couple of months back with a discussion on Generative AI (Gen-AI). It generated some discussion that was interesting; this author also talked on the subject with consulting clients, prospects, peers, friends and family. An interesting common perception emerged: “Gen-AI is all and only about text and image generation.” ChatGPT and DALL-E created enough sensation to become the sole “spokespersons” for Gen-AI. This is akin to catching a tiger by its tail so that we will look at the REAL business value of Gen-AI; we will look at real-life use cases that are either already life or are WIP and their impact on the core business.
Before looking at use cases, let us try to understand Gen-AI. If we were to look at traditional IT jargon, Gen-AI is Decision Tree, Recursive Loop, and Data Management on steroids, and then SOME MORE. The point being made is that there is potential for business applications. In fact, Gen-AI is built around the concept of CREATIVITY; neurologically speaking, ‘creativity’ is the process of making connections that are not obvious and may even appear weird. The difference between human creativity and Gen-AI is that the latter is Explainable.
Two more points are worth noting before we look at the live examples. Firstly, Private datasets are the way to go; even if one uses public data (in addition to internally generated data), it should be brought (copied) into the private dataset. The negative stories of the NY Times Food Reporter’s Thanksgiving Di.nner Menu or the Washington Post reporter being told of mankind's destruction methodology were based on public domain data. This data is full of errors, omissions, fraud, and bias, so the result will not be reliable for businesses.
Which brings us to the second key point. Gen-AI’s full power is exploited alongside another technology that generates relevant and specific data. Let us now look at a few use cases.
GE Aviation started with the objective of reducing unplanned downtime for its jet engines. It installed IoT sensors on jet engines, and Gen-AI uses this data to predict the need for maintenance. This has allowed GE to schedule the maintenance activity proactively. GE Aviation has been able to reduce its unplanned downtime by 20%.
Maersk wanted to make its shipping operations more efficient. So, it created Digital Twins of its ships and supply chains. Gen-AI simulated the performance under different conditions, including weather, traffic, demand, etc. By predicting the problems before they occur, Maersk has improved its on-time delivery rate by 3%.
Nvidia used Gen-AI to power autonomous vehicles. Edge computing collects data from vehicle sensors like cameras, radar, light detection, ranging, etc. Gen-AI uses this data to make decisions about vehicle control, and the edge devices make these decisions in real-time, right at the spot they are to be implemented. Nvidia also uses Gen-AI in another unrelated application. It uses Gen-AI to train and deploy new AI models for spatial computing. Developers use its Omniverse platform to train and test new spatial computing models.
Magic Leap is another company using Gen-AI with spatial computing. Integrating these into its Augmented Reality platform adds realistic 3D objects and environments for real experiences. This platform is still under development, though initial versions are already available and deployed for many uses, including spatial audio and a brush to draw across multiple devices from multiple locations.
Stratasys was searching for newer ways to manufacture aerospace parts. It uses Gen-AI algorithms to generate new aerospace parts designs (that are optimized for weight and strength). The designs are then sent to the Additive Manufacturing system for prototyping and manufacturing. The new parts are lighter and stronger and cost less.
These are just six of the dozens (if not hundreds) of real-life examples. All these beg the question: is simple text generation an underutilization of Gen-AI? Not really. This author is currently working on a purely text-based consulting assignment, which will be on a private dataset. This system will allow a team of just ten customer service executives to answer 150,000 questions on operating 1500 variations (brand, size, model, etc.) of all household appliances. It will ALSO allow an on-site service technician to learn about the installation or repair of these appliances, all in real-time. These technicians will mainly be in over 500 Tier 2, 3, and 4 cities nationwide. Just to give the readers a perspective, this company, Serviz, currently finds a service technician in these 500+ cities who knows the particular brand of the appliance to be serviced; the new system will make the service technician brand-neutral.
The conclusion from all these examples is that the IT Departments should stop selling themselves short and work with business functions to exploit this easy and economical tool.
Akash Jain is a Former CEO, Mastercard JV & Former CTO of Reliance Retail Finance.
Image Source: Freepik
Add new comment