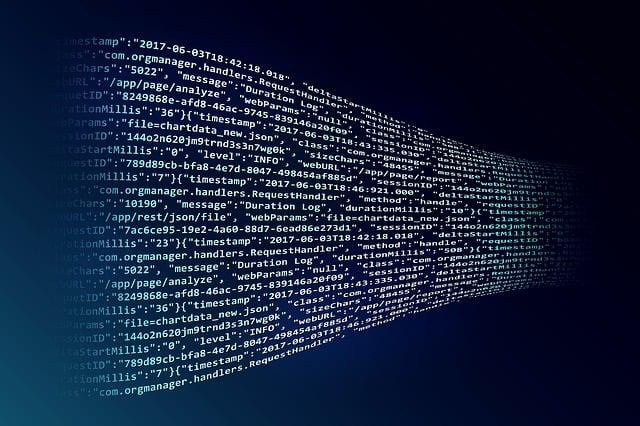
In 2021, enterprises reportedly spent an astounding $215 billion on big data and business analytics solutions, and IDC analysts predicted a 19% increase in this spending in the year 2022. With the increasing spending, the need for experts in data analytics also grew in 2022. In the year 2023, it is expected that almost 90% of organizations would consider information as a ‘vital enterprise asset’ and analytics as an ‘important capability’.
However, the world of analytics and data is always evolving. Technologies that provide quicker and more accurate access to information are continually being developed. Here is the rundown of the key trends that will have the biggest impact on how we use data and analytics to fuel business success in 2023.
Big Data Fabric, DataOps, and Data Contracts
These are the concepts that will gain popularity in the year 2023.
With the help of automated data ingestion, curation, discovery, preparation, and integration; big data fabric is a platform concept that aims to accelerate business insights. Large enterprises produce huge data, very fast, and from several systems. Such a concept that delivers faster insights will become quintessential for these large enterprises.
DataOps is more of an architectural framework and approach, that might be viewed as a part of the bigger ‘data fabric’ platform. The ideas of agile development, DevOps, and statistical process control are all combined in this. It has been developed from the DevOps concept used in software development and will be a go-to approach for large enterprises when it comes to obtaining faster insights.
Data contracts are becoming increasingly important as these describe the data to be exchanged and the way it must be used. Data contracts are giving confidence to the data experts in managing their data pipelines and defining the standards.
Data as a Service (DaaS)
Industrializing data and developing a modular, reusable data engine are two cornerstones for attaining a high speed-to-market for analytics and reporting objectives.
DaaS will become a critical pillar that would enable customers to drive complicated analytics transformation journeys by involving businesses to play a larger role from the very beginning of the transformation projects.
Businesses may benefit from adopting DaaS in several ways because it keeps their data management processes agile, reduces the time it takes to get insights, and enhances the dependability and integrity of their data. DaaS enables enterprises to build, deploy, and manage modular, reusable data engines that may be used as a foundation for attaining high speed-to-market for analytics and reporting needs. It also covers the whole data analytics lifecycle.
Utilising AI for MetaData Management
MetaData management, which is a framework for cataloguing data assets within your organisations throughout their existence, is not a new idea. But this idea is expanding beyond its traditional confines of compliance and risk management and is now enabling businesses to improve on crucial performance areas, since managing data in large corporations is becoming more difficult.
It is crucial to have a method for creating metadata at a much quicker pace since data is rapidly being extracted from several sources with increased velocity and volume and made available in a variety of formats.
Here, utilising AI/ML will become increasingly important and will be a key differentiator.
Augmented Analytics
The idea of augmented analytics is to make AI/ML practical for data preparation, insight generation, and insight explanation. Traditionally, the insight creation process has been quite ad hoc, and it required a large team of experts to interpret the information and data. Thanks to augmented analytics, data exploration and synthesis is now considerably faster.
This will become crucial for large organisations that produce data from various systems in a variety of formats. Instead of merely counting beans, analytics specialists would focus more on delivering business values using advanced analytics.
Democratisation of AI/ML in finance function
There has been a huge application of AI/ML in different domains, such as marketing, sales, supply chain, etc., in the last few years.
However, finance functions of the enterprises are the slow adopters of AI/ML, due to the reason that a high level of interpretability along with accuracy is required with finance data. The results of a finance analytics project are often referred to directly by high-level leadership to make strategic decisions that can significantly alter the future course of an organization.
But business leaders are now realizing that AI/ML applications can significantly help the finance teams in providing avenues where profit can be maximized, risks can be minimized, new investments can be made, etc. Finance functions of large enterprises will increasingly deploy AI/ML in the year 2023, with applications of all sorts of techniques, from highly interpretable statistical techniques to very accurate deep learning techniques.
- The author is Principal Data Architect, Aays
Add new comment